Research News: Initial Study of Reinforcement Learning Algorithms in Cyber-security Simulations
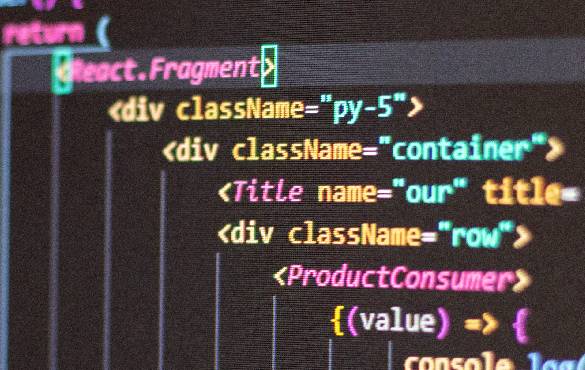
Alberto Acuto, Data Scientist at the Signal Processing Group, provides an overview of his research paper; Initial Study of Reinforcement Learning Algorithms in Cyber-security Simulations
Summary
With the development of novel technologies and more complex infrastructure that we collectively use and live in daily, such as work environment, banking systems or healthcare the need for effective and reactive methods to defend the networks from harmful intent is more than ever requested. In this context, the exploration of novel algorithms and methods to develop autonomous and resilient agents that can detect and react to external and malevolent aggression is a necessary step that many agencies and governmental bodies have identified as an achievable goal in the near future.
Importance of the research
To explore and validate the development of autonomous agents, the defence science and technology laboratory (DSTL) in the UK developed a network simulator, Yawning Titan, which simulates a malicious agent that tries to take over a network and a defending agent tries to react and respond to the threats. This software aims to create a system where it is easy to explore the performances of reinforcement learning algorithms in training the defending agent to react more effectively to the enemy's actions. The interest in this work came from the first test of the possibilities of the software, which was publicly released, in its first version, in the summer of 2022 (now 2.0.1b) in exploring how popular reinforcement algorithms (such as proximal policy optimisation, known as PPO) were effective in the deployment of an agent in a set of different networks of different size (from few to hundreds of nodes) and scenario.
In literature, the focus and testing ground of many RL algorithms is in a game-like environment, where these algorithms teach an “agent” how to make relevant decisions to maximise the score of such a game and ultimately win. In the context of cyber-defence, the interest was to understand and quantify how the trained agents were resilient in reacting in varying environments such as networks with existing compromised conditions (as nodes disconnected from the network or nodes already compromised), different shapes of networks (fewer or more connections between nodes), and how complex would be to update such agents to perform with less performance degradation in these cases. We measured the performances of some agents trained with different algorithms in realistic network environments and validated that these agents tend to react quite effectively to the presence of a network different from the training ground. More interestingly, the degradation of performances looks minimal compared to the overall performance obtained in a more “known” environment.
What comes next?
This work opened and explored the potentiality of the Yawning Titan software, given the chances of setting up different networks and modifying the status of the starting network simply. Different single and multi-agent algorithms can be explored, as well as challenging the agents using a different set of simulation rules. With broader tests, it will be possible to have a panoramic view of the options available and measure effectively how, also in the cyber context, the deployment of autonomous agents to defend real networks is not so far away from reality.
Alberto had his paper accepted for Dstl AI Fest 5 2023.