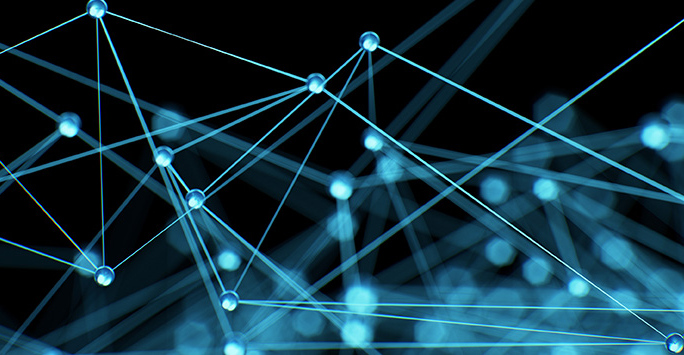
A preprint submitted to Arxiv and submitted for peer-review, demonstrates how we are configuring the proposal for the No-U-Turn Sampler (NUTS) into a Sequential Monte Carlo (SMC) sampler.
The research
NUTS is a popular Markov Chain Monte Carlo (MCMC) algorithm for Bayesian inference problems due to its efficient exploration of continuous probability distributions. However, NUTS when used for MCMC is difficult to parallelise. SMC, an alternative approach to Bayesian inference, on the other hand is readily parallelisable. This paper demonstrates how we can utilise the benefits of SMC while also using the modern developments of MCMC to produce an even better sampling method. SMC samplers also have an additional benefit over MCMC in that they have an additional tuning parameter, namely the L-Kernel. This paper demonstrates how a recent paper co-authored by the Signal Processing Research Group fits into this new work to present a framework of how a near-optimal L-kernel can be derived for a NUTS based proposal.
Why it matters
This is one more stepping stone in the year of the SMC sampler. The group is breaking new ground on several fronts of SMC development. The work presented here could lead the way to showing how SMC samplers provide strong scaling using Hamiltonian based proposals. This would be a game changing outcome and would allow scientists and engineers around the world to run their models and data analysis for a fraction of their current runtime on high performance computing architecture.
We’re thinking
While we do demonstrate how a near-optimal L-kernel fits into an SMC sampler with a Hamiltonian based proposal, this approach only works well at low dimensions. To be generalised to higher dimensions requires some more work. This is a current focus within the group and the Distributed Algorithms CDT.
Access the preprint and send your thoughts to Lee Devlin ljdevlin@liverpool.ac.uk