Research News: Bayesian Detection with Long Time Integration
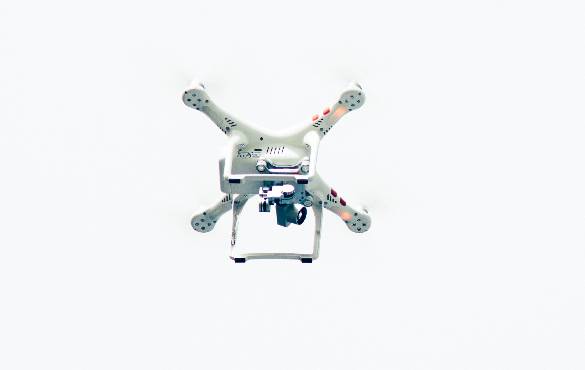
Murat Uney, Lecturer in Signal Processing, provides an overview of his recent paper publication relating to the proposal of an algorithm which simultaneously estimates the unknown trajectory of craft using Bayesian detection test for long-time integration.
Summary
This work addresses processing challenges in the detection of dim and manoeuvring objects, such as drones, stealth aircraft or rigid inflatable boats (RIB) with radars. Radars detect objects by probing the environment with electro-magnetic pulses and seeking for reflections of these pulses in their receiver antenna signals. Dim objects induce small reflection signals which are easily buried under noise, making their detection difficult. Collection of evidence in the receiver signals for a long period of time (i.e. long-time integration) makes detection possible, however, it is complicated by the manoeuvres of these craft and its unknown trajectory. We propose an algorithm which solves the long-time integration problem in radar signal processing by simultaneously estimating the unknown trajectory of craft and performing a Bayesian detection test.
Importance of the research
One alternative for long-time integration is using a bank of filters each of which matches a possible trajectory. The number of filters needed, however, grows combinatorially with the integration time practically limiting it with small numbers. Our approach allows much larger integration time by sequentially estimating the trajectory of a craft, and adapting the processing of the received signals accordingly.
The proposed algorithm processes coherent baseband signals, i.e. complex in-phase and quadrature-phase signals, and can be tailored for a wide range of radar configurations, e.g. different pulse shapes, pulse repetition intervals, receiver aperture, and working with non-coherent signals. This versatility makes the approach applicable for a wide range of radar sensing applications including maritime and air situational awareness.
What comes next?
Future work will extend the underlying model to estimate a larger set of signal parameters jointly and look at using efficient Bayesian computations such as the No-U-Turn Sampler (NUTS). These steps will improve the robustness of the algorithm widening its range of applications.
This paper is published in the IEEE Signal Processing Letters and will be presented at the 2023 edition of the IEEE ICASSP.