Research News: Probabilistic Decision Trees for Predicting 12-Month University Students Likely to Experience Suicidal Ideation
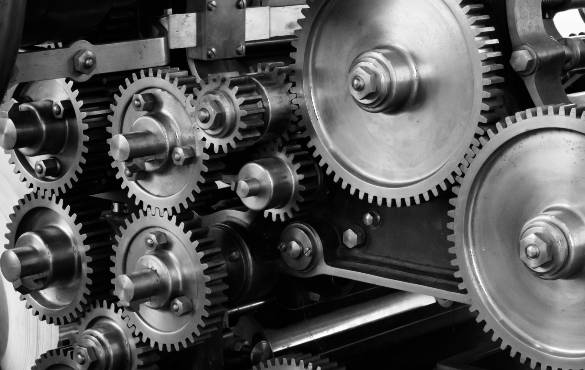
Efthyvoulos Drousiotis, Year 3 PhD student at the CDT, provides an overview of his recent research relating to Probabilistic Decision Trees for Predicting 12-Month University Students Likely to Experience Suicidal Ideation. The paper was published on AIAI2023.
Summary
Environmental stressors combined with a predisposition to experience mental health problems increase the risk for SI (Suicidal Ideation) among college/university students. However, university health and wellbeing services know little about machine learning methods and techniques to identify as early as possible students with higher risk. We developed an algorithm to identify university students with suicidal thoughts and behaviours using features universities already collect.
Importance of the research
The relationship between Suicidal Ideation (SI) to either attempting or committing suicide is complex. For example, the assumption of a straightforward ’linear progression’ from SI to action is not empirically supported. Given this complexity, locating individuals who might benefit from enhanced support and early intervention for both mental health problems and features associated with suicide may be logistically and practically more appropriate.
Early identification of at-risk students is important for effective suicide prevention and allows universities to refer students to appropriate well-being, counselling, and pastoral support. Moreover, most of the papers in the literature tend to report analyses conducted on samples that contain relatively small amounts of data, with some large inconsistencies in sample sizes across previous studies.
To address this issue of inconsistent and relatively small sample sizes used in past research, we conducted machine learning-based analyses on a sample of 50,307 students from the American College Health Association (ACHA) survey to provide a more robust and reliable understanding of the predictors of suicidal ideation in university students. To achieve this aim, we conducted two separate analyses.
Firstly, a machine learning model featuring data that universities are likely to collect as part of their normal operations was fitted to the data (e.g., information about the student’s general health). Secondly, we tested a more comprehensive model featuring this information plus a variety of variables from the ACHA survey implicated in suicidality (e.g., social connectedness variables) which universities may not normally collect from their students. We ran these two models to investigate which offers the more robust and accurate predictive model of suicidal ideation in university students and identify possible important vulnerability factors for suicidality in students that universities may need to collect in the future. A scalable and transparent state-of-the-art machine learning algorithm, a parallel MCMC Decision Tree, processed the models.
What comes next?
Our approach can potentially help universities quickly identify and provide early interventions targeting students with these suicide-risk factors. This study, though, has some limitations we are aiming to address in future research. The study focused only on SI and not suicidal behaviours, as we should note that the results may not be generalisable to students in other countries.