Research News: Inference of Stochastic Disease Transmission Models Using Particle-MCMC and a Gradient Based Proposal
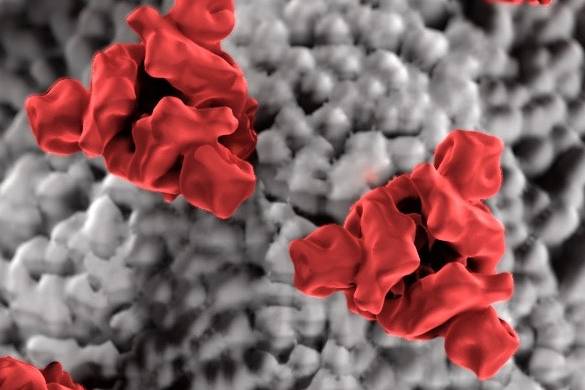
Conor Rosato, research associate at the Signal Processing Group, introduces his latest research paper.
Summary
A section of our research group collaborated to devise a method to answer a complex problem previously described as not being possible. Applying this method to disease specific models allows us to efficiently infer the model parameters with more accuracy in less computational time than the previous state-of-the-art method. Think of the parameter that best describes the data as being at the top of a hill. We need to efficiently climb the hill to reach this parameter. Our method uses gradient information to navigate to the top of the hill in an informed way while the previous state-of-the-art method navigates the hill randomly.
Importance of the research
I recently finished a 6 month secondment at the United Kingdom Health Security Agency (UKHSA) to help with the joint effort of modelling COVID-19. During the secondment, the need for robust algorithms that could efficiently infer the parameters of disease models became apparent. Along with my supervisor at the UKHSA, Jasmina Panovska-Griffiths, we applied and compared this novel technique to the previous state-of-the-art and found we outperformed it in terms of accuracy and run time. Although the results are for a simple model with simulated data, we have provided a proof of concept that could easily be extended to include more complex models with real data. While working at the UKHSA I met people from both academia and industry that were keen to collaborate in improving these techniques further.
What comes next
The next steps involve producing an easy to use software package that makes use of existing probabilistic programming languages to efficiently estimate the parameters of stochastic disease models.
This article belongs to the CDT's Fusion 2022 series. Please review our other Fusion conference paper overviews.