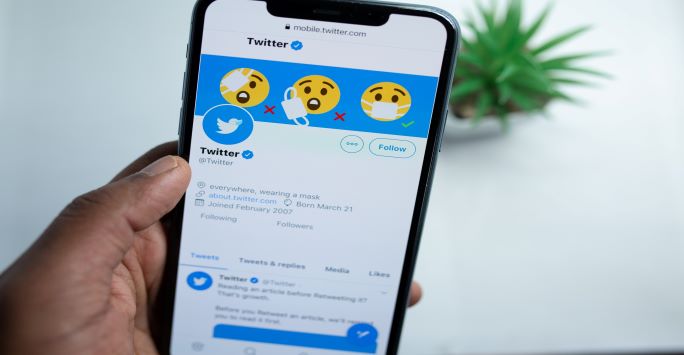
Watching your favourite movie. Selling that old armchair. Or getting through a new bout of illness – whatever you want to talk about, social media is now a firmly mainstream way to do it. And with so many users, that’s a lot of information. However, we still haven’t tapped into the full potential of these data for scientific research, particularly for how they might shed light on the health needs of local communities.
I’m a computational biologist interested in how we can better use data and dynamic ‘AI’ to understand infectious diseases and their transmission. One of the things I’m particularly excited by is text mining, a field that aims to generate useable scientific data from all kinds of texts. For example, I’ve previously used social media data to estimate sentiment towards vaccination in the UK and to differentiate scientific and public reactions to preprint research.
Recently I’ve been thinking about a new question: could we use text data from social media as a form of surveillance for infectious diseases?
Through an award from the University’s Wellcome Institutional Strategic Support Fund, I aimed to explore this and develop a new collaboration with the NIHR Health Protection Research Unit in Gastrointestinal Infections, who are particularly interested in how COVID-19 may have affected gastrointestinal disease.
I wanted to share some ideas that I’ve learned from this project on why health researchers should consider social media sources. I focused on Twitter as it’s a reasonably standardised and practical data source to use. Researchers have used Twitter data to track views on infectious diseases for a long time, but now it’s easier than ever (and free!) for academics to obtain access to Twitter data for research purposes.
It is possible to investigate a wide variety of questions with this concise yet expressive textual data source such as:
• identifying concepts (‘Are there possible adverse reactions associated with this drug?’)
• visualising time trends (‘Have patterns of seasonal allergies changed over the past few years?)
• investigating co-occurrences (‘Are pet owners at greater risk of this infection?’)
I was interested in capturing trends in symptoms reported on Twitter, because the first thing many opt to do when they feel unwell is share what they’re experiencing. A strength of social media is that it’s essentially real-time reporting so it can provide early warnings of emerging health threats such as predicting when seasonal influenza will begin. These early warnings can in turn forecast healthcare demand e.g., emergency hospital admissions, allowing authorities to better pre-empt where to concentrate resources.
Another advantage is that social media may capture information on individuals and communities that aren’t well represented by conventional surveillance. This was particularly important for us, as data from COVID-19 testing has drastically reduced in the past year. Although Twitter certainly isn’t unbiased in the kinds of users represented on the site, it might be able to ‘fill in’ some demographic gaps that would be otherwise hard to determine from medical records, like particular occupations or roles such as carers. This opens up exciting potential for findings to inform more tailored public health initiatives for example, campaigns for tobacco control could target social phenomena identified from tweets.
An interesting observation was that tweet texts often challenge you to widen your thinking on search terms. Funnily enough, virtually no-one tweeted “I have acute rhinitis” to say they had a runny nose. This is sometimes not for the feint hearted as you can get unfiltered opinions within tweets, including strong language(!) It’s also worth acknowledging the inherent bias that comes with using English-language tweets only.
Finally, Twitter data sits in a strange space between revealing often quite personal information yet being open and publicly available. Where possible I’d recommend anonymising account names in any data you extract from Twitter and not retaining the original text of the tweets after all necessary processing. There are many more common questions about ethical use of Twitter data than I can discuss here, but must be considered if you’re planning a new project using social media data.
If you’ve been inspired to use this powerful data source, a good place to start with practical tools is this tutorial from the UK Data Service which introduces various packages to handle tweet data in Python (a step-by-step video introduction from the creator, Joe Allen, is also available).
It's been really exciting to consider how people talk about their symptoms on Twitter for better disease surveillance through the support of the Wellcome ISSF. No doubt this contemporary source of information will become a part of ‘smarter’ future healthcare systems - systems that truly reflect the targeted local needs of residents and adapt as these needs change over time.