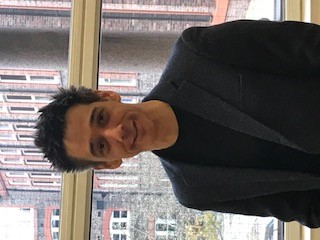
Professor Daniel Joyce
Professor of Connected Mental Health Primary Care & Mental Health
- Work email D.Joyce@liverpool.ac.uk
- Personal Websitehttps://www.danwjoyce.com
- ORCID0000-0002-9433-5340
- About
- Research
- Publications
About
Personal Statement
I'm a psychiatrist and data scientist interested in how we can make use of data-driven technologies to better understand mental illness. My work looks at how we can use methods from statistical- and machine-learning in prosaic ways to deliver actionable insights to patients and clinicians. Topics I have a particular interest in include a) defining what transdiagnostic phenotyping means and how it can be achieved using clinical data that may be at best, first-approximations (or proxies) for traditional diagnostics and syndromes b) unobtrusive and low-burden measurement and tracking of clinical and health states using technology c) how to capture aspects of clinical decision making so that data-driven technology (like artificial intelligence) can be aligned with clinical practice.
I spend much of my time working on delivering two work packages for the Mental health Research for Innovation Centre -- specifically, Innovative Therapies for Mood Disorders and the Mental Health Avatar.
My colleagues and I have a number of active projects, including:
- natural language processing (especially large language models) for capturing signatures of phenotypes in electronic health data (e.g. difficult-to-treat depression) including how synthetic data can be meaningfully defined and generated to support development of these signature-detection methods
- when algorithms are used to assist clinical decision making, how can uncertainty be quantified and presented to users of these tools
- intrinsically-interpretable machine learning for assisting clinical decision making e.g. using decision-tree based representation and algorithms
- how to make the measurement and processing of clinical multivariate time-series data (passive sensing as-well-as active experience sampling) useful to clinicians and patients (i.e. going beyond anomaly detection such as flagging or predicting "relapse" events)
- socio-technical approaches to mitigating bias in data and algorithms, particularly the problem of a) mental health data fidelity for under-represented groups and b) defining the appropriate (re)use-cases to ensure data-driven technology doesn't further embed bias or inequity because data is of poor quality or has been re-used outside of it's intended purpose
Please do reach out if you have an interest in working with us on these areas. Note that we are accepting expressions of interest from potential Ph.D. students but we have limited funding available currently.