Development of spatiotemporal statistical methods for anomaly detection allows the detection of regions or seasons in which symptoms or diseases of interest are unusally prevalent. This research uses data from veterinary practices and diagnostic laboratories participating in SAVSNET, both the syndromes selected by the veterinary surgeon and also text mining methods. This research is closely linked with the work being done at the University of Bristol and the Animal Health Trust which focuses on disease outbreaks and setting disease outbreak thresholds.
Charlotte has been working closely with the University of Liverpool and the University of Manchester in exploring SAVSNET data and the syndromes recorded. Linking with the University of Bristol and Animal Health Trust, discussions have begun regarding thresholds and relaying information back to stakeholders.
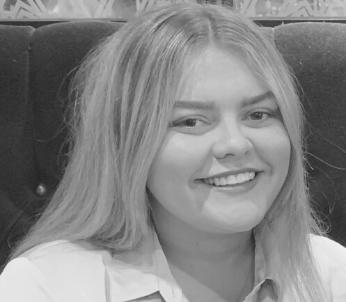
My work will be analysing real-time veterinary data at a national level which allows us to detect anomalies and therefore will aid in trying to predict any outbreaks in future.
Charlotte Appleton
Back to: Small Animal Veterinary Surveillance Network (SAVSNET)