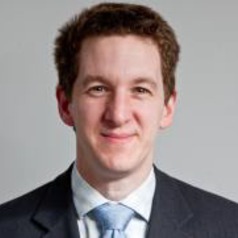
Professor Simon Maskell MEng, MA, PhD, FIET, CEng
Professor of Autonomous Systems Electrical Engineering and Electronics
- +44 (0)151 794 4573
- Work email S.Maskell@liverpool.ac.uk
- Personal WebsiteSimon Maskell's personal website
- About
- Research
- Publications
- Teaching
- Professional Activities
Research
Big Data and Autonomous Systems
Big Data is the use of distributed computational resources (i.e., big computers) to tackle problems that involve too much data to fit on a single computer or data arriving at too high a rate for a single computer to ingest and/or process. Frameworks (e.g., Hadoop, Spark, Storm, MPI, OpenMP) exist to handle some of these problems. Research is focused on solving problems that such frameworks alone do not address. Typically such problems involve processing ambiguous, incomplete and conflicting data to extract information pertinent to human decision making.
Autonomous Systems are systems that ambiguous, incomplete and conflicting data to extract information pertinent to automated decision making (e.g., by a robot). From an algorithmic perspective, the distinction between Big Data and Autonomous Systems relates to who makes the decision.
Bayesian Statistics (including Numerical solution methodologies)
Bayesian statistics is a powerful mathematical approach that uses an explicit articulation of prior knowledge to turn data into the information needed for decision making. The decisions can be made by people (e.g., in the context of Big Data) or by machines (e.g., in the context of Autonomous Systems). The focus of Bayesian statistics is primarily on articulating experts' understanding in a way that allows statistics to be used to describe and propagate uncertainty.
Often the mathematics involved in making Bayesian statistics a reality demands the use of Monte Carlo simulation. Such "numerical methods" (e.g., Markov Chain Monte Carlo (MCMC), particle filters and Sequential Monte Carlo (SMC) samplers) are widespread. Research focuses on efficient simulation, particularly in challenging scenarios (e.g., involving parameters that are high-dimensional).
Tracking and Data Fusion
Tracking is the task of combing information in the context of an incoming data stream relating to each of many objects (e.g., aircraft observed by a radar). Data fusion involves combining information in the context of data from multiple sensors. Both demand careful application of Bayesian statistics. Research focuses particularly on the use of particle filters, a high performance algorithm that has been demonstrated to be a very effective way of extracting pertinent information in the context of tracking and data fusion problems.
Research Grants
Manifold Embedding for X-ray Image Object Recognition
HOME OFFICE (UK)
November 2016 - March 2019
Fighting International Internet Paedophilia – Phase Two.
HOME OFFICE (UK)
April 2015 - March 2017
Ways of Being in a Digital Age: A Systematic Review
ECONOMIC AND SOCIAL RESEARCH COUNCIL
August 2016 - October 2017
Multi source automated NSIF generation.
EADS INNOVATION WORKS UK
March 2014 - September 2017
WEB-RADR
EUROPEAN COMMISSION
September 2014 - August 2017
Bayesian Analysis of Competing Cyber Hypotheses
ENGINEERING & PHYSICAL SCIENCES RESEARCH COUNCIL
October 2014 - October 2017