Paper published: outlier management in growth data
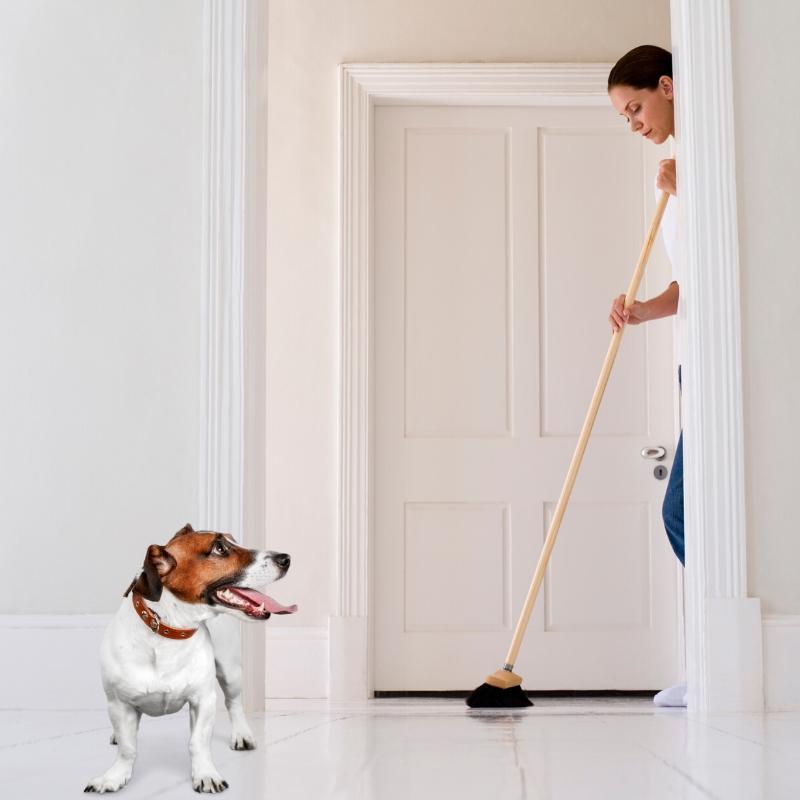
The latest paper using SAVSNET data, led by Charlotte Wooley at The Roslin Institute (University of Edinburgh) has been published in Plos One.
With other organisations, SAVSNET supplied data relating to the weight of dogs for a novel algorithm to be developed for data cleaning.
All data are prone to error and require data cleaning prior to analysis. An important example is longitudinal growth data, for which there are no universally agreed standard methods for identifying and removing implausible values and many existing methods have limitations that restrict their usage across different domains.
- A decision-making algorithm that modified or deleted growth measurements based on a combination of pre-defined cut-offs and logic rules was designed. Five data cleaning methods for growth were tested with and without the addition of the algorithm and applied to five different longitudinal growth datasets: four uncleaned canine weight or height datasets and one pre-cleaned human weight dataset with randomly simulated errors.
- Prior to the addition of the algorithm, data cleaning based on non-linear mixed effects models was the most effective in all datasets and had on average a minimum of 26.00% higher sensitivity and 0.12% higher specificity than other methods.
- Data cleaning methods using the algorithm had improved data preservation and were capable of correcting simulated errors according to the gold standard; returning a value to its original state prior to error simulation.
- The algorithm improved the performance of all data cleaning methods and increased the average sensitivity and specificity of the non-linear mixed effects model method by 7.68% and 0.42% respectively.
- Using non-linear mixed effects models combined with the algorithm to clean data allows individual growth trajectories to vary from the population by using repeated longitudinal measurements, identifies consecutive errors or those within the first data entry, avoids the requirement for a minimum number of data entries, preserves data where possible by correcting errors rather than deleting them and removes duplications intelligently.
- This algorithm is broadly applicable to data cleaning anthropometric data in different mammalian species and could be adapted for use in a range of other domains.
Access the paper here