Antimicrobial resistance (AMR) is a critical challenge for human and animal health that requires a coordinated endeavour across the disciplines of clinical and data science. The project is a collaboration with Dr Peter-John Noble at the SAVSNET group utilising their dataset which has amassed over 8 million veterinary electronic health records. Previous works from this group have arisen from strategies such as using regular expressions, although this has been successful for highly specific targeting of a singular disease or condition it is not appropriate for a more wider analysis which we are wanting to complete here. Sean has joined Dr. Noura Al-Moubayed lab within the Innovative Computing Group at Durham University to achieve this integration of novel machine learning strategies and distilling them into the emerging field of veterinary bioinformatics. This project seeks to embody this interface to recognise the features and signals that might be available in a large companion animal clinical records dataset and to develop and apply cutting-edge machine-learning methodologies to derive important insights.
PUBLICAITONS from this work
PetBERT: automated ICD-11 syndromic disease coding for outbreak detection in first opinion veterinary electronic health records. Scientific reports
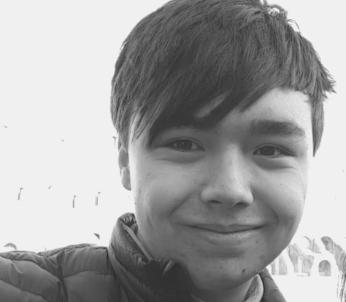
Antimicrobials are an invaluable tool that has changed the course of history, however the return to a pre-antimicrobial world is a not-so-distant reality. Veterinary practitioners are as equally responsible in maintaining good antimicrobial stewardship and I would hope this project can increase awareness and reduce unnecessary prescriptions
Sean Farrell, PhD postgraduate researcher
This research is funded by the BBSRC Doctoral Training Partnership
Back to: Small Animal Veterinary Surveillance Network (SAVSNET)