The use of metrics can provide valuable insights into aspects of research in some disciplines if used critically. However, when metrics are used in the wrong context, it can be problematic for researchers and research progress. Inaccurate assessment of research might become unethical when metrics take precedence over expert judgement, where the complexities and nuances of research or a researcher's profile cannot be quantified.
Metrics Bites
These short videos give an introduction to metrics. Select the topic you're interested in, or watch all videos in order to get the full picture. Captions are available.
What are metrics? |
Bibliometrics introduction: |
Alternative metrics introduction: |
---|---|---|
Bibliometrics example:
|
DORA explained: |
Alternative metrics example:
|
Metrics - Levels and providers
The below graphic gives a visual representation of the most commonly used metricsm their pros and cons, and whether to avoid them for assessment purposes. Click on the image to enlarge it.
Journal-based Bibliometrics
Journal-based metrics should not be used as they refer to the venue of publication rather than the individual output, and are not a good indicators of the quality of the research or its impact. Additionally, Journal metrics do not well reflect new/emerging fields of research.
JIF = Journal Impact Factor (Clarivate Analytics, Web of Science)
Journal Impact Factor is calculated by taking the number of citations for that journal in the last two years and dividing it by the number of citable items in said journal. It does not reflect the true number citations of each output.
It is a commercial product owned by Clarivate Analytics, the data are sources from Web of Science. JIF was invented by Eugene Garfield, a librarian and bibliometrician from the US, in order to advise libraries which journals to subscribe to. In the absence of more suitable indicators, it also became a proxy for assessing the quality of research. However, this approach is very problematic and has been challenged in the last decade by the research community, which is calling for change.
The major flaws of using JIF include:
- Citation distributions within journals are highly skewed. JIF is a simple average, which is highly susceptible to outliers. By publishing a few papers that attract a large number of citations, the results can be skewed by pushing up the mean.
- JIF is Field-specific. The amount of citations received in different fields vary significantly. For example, Physics papers often refer to particular scientific methods or theories that have been used or considered and thus include a lot of citations. Whereas in History, researchers tend to describe the theory or method in more detail rather than cite others and therefore in general have fewer citations. This becomes very problematic when comparing institutions with varying research units across disciplines.
- JIF can be manipulated by editorial policy, self-citations and citation 'cartels'. For example, editors can boost the citations by:
- asking authors to cite articles from the same journal
- deliberately publishing more citable paper types such as review article
- reducing the number of "citable items" in the JIF calculation
- Data used to calculate JIF are not transparent nor openly available.
Cons:
- The JIF does not exist for all journals, all disciplines, or even outside any publications that are not indexed by Web of Science.
- Citation distributions within journals are extremely skewed: the average number of citations an article in a specific journal might get can be a very different number to the typical number of citations an article in a specific journal might get.
- The JIF is nothing more than the mean average number of citations to articles in a journal, and thus highly susceptible to outliers.
- Journal metrics do not well reflect new/emerging fields of research.
CiteScore (Elsevier, Scopus)
CiteScore is a metric provided by Elsevier, data are taken from the Scopus database. It is calculated the same way as JIF with the exception of taking into account the previous 3 years instead of 2. CiteScore suffers from the same flaws as JIF.
Cons:
- Citation distributions within journals are extremely skewed – the average number of citations an article in a specific journal might get can be a very different number to the typical number of citations an article in a specific journal might get.
- As with JIF, the CiteScore is nothing more than the mean average number of citations to articles in a journal, and thus highly susceptible to outliers.
- Journal metrics do not well reflect new/emerging fields of research.
Eigenfactor (Clarivate Analytics, Web of Science)
Eigenfactor is a rating of the total importance of a scientific journal within a selected network of journals, similar to Google page ranking. Citations from highly ranked journals are weighted to make a larger contribution than those from poorly ranked journals. The Eigenfactor is based on citations made in a given year to papers published in the prior five years and eliminates self-citation. The calculation includes a complex algorithm for the determination of eigenvectror centrality, which defines the journal's percentage of the total influence in the network. For a standard user, the computational calculation is difficult to verify.
Cons:
- Aims to measure the ‘total influence’ of a journal. The subject field, quality and reputation of the journal directly affect the value of a citation.
- Favours long-running journals as the numbers are added, not normalised.
Article Influence Score (Clarivate Analytics, Web of Science)
When the Eigenfactor is adjusted for the number of papers published in each journal, it is called the Article Influence Score.
Cons:
- Is calculated by dividing the Eigenfactor Score by the number of articles of a journal and normalizing the scores so that the average score equals 1.
- Does not consider different citation practices in different fields.
- Because it only uses averages to measure articles, and despite its name, it still is a journal-level metric.
SJR = Scimago Journal Rank (Elsevier, Scopus)
SJR is a prestige metric similar to Eigenfactor calculated by a similar computational algorithm. The main difference is that SJR does not eliminate self-citations and uses 3-year window of citations.
Cons:
- Citations are weighted based on the source that they come from. The subject field, quality and reputation of the journal directly affect the value of a citation.
- The SJR is a journal-based metric and thus the metric applies to the place that an output is published rather than the merits of the output itself.
- Journal metrics do not well reflect new/emerging fields of research.
SNIP = Source normalised impact per paper (Elsevier, Scopus)
A journal's SNIP is CiteScore divided by the citation potential. Citation potential is a reflection of how likely paper is to be cited in the given field.
SNIP citations are normalised to correct for differences between scientific fields; thus it removes one of the main flaws of JIF and CiteScore. However, it is still a journal based metrics with all the remaining limitations.
Pros:
- SNIP corrects for differences in citation practices between scientific fields, thereby allowing for more accurate between-field comparisons of citation impact.
- SNIP comes with a 'stability interval' which reflects the reliability of the indicated - the wider the stability interval, the less reliable the indicator.
Cons:
- Despite its name, and although consideration is taken to correct for differences in fields, the SNIP is still a journal-based metric and thus the metric applies to the place that an output is published rather than the merits of the output itself.
- Journal metrics do not well reflect new/emerging fields of research.
Metrics based on articles citation count
Raw citation count
A common assumption is that a high amount of citations means that a paper is a good piece of research and/or has had a positive impact. However, this is not always the case for the following reasons:
- The citations can be negative, positive, neutral or even quite random and unless we examine all the references, we cannot know what their intent is
- Citations are not reviewed and removed in case some articles have been retracted.
- Some authors deliberately cite their own or colleague's articles even if the relevance is rather questionable.
- Certain types of articles tend to get more citations than others – for example, reviews are more cited than a traditional research journal article
All the above leads to skewing of the numbers.
Pros:
- A simple-to-read measure of attention when comparing outputs of the same type and age within the same field.
Cons:
- Citation practice varies across fields; the same number of citations could be considered low in one field e.g. immunology but high in another e.g. maths.
- Certain output types such as Review Articles will frequently be more highly cited than other types.
- As an example of how citation counts can be artificially inflated, the paper "Effective Strategies for Increasing Citation Frequency" lists 33 different ways to increase citations.
h-index
h-index is the number of publications for which an author has been cited at least that same amount of times. So if a researcher had an h-index of 10, it would mean they would have at least 10 papers that have been cited 10 times.
There are a number of issues with the h-index.
- It can be manipulated by self-citations. It has been shown that men tend to self-cite more than women.
- It does not account for disciplinary differences.
- It favours senior researchers who get their name by default on articles published by their junior colleagues.
- It is based on productivity, and therefore it favours those who have been in their career a long time and haven't taken a break. It discriminates early career researchers, women, part-time researchers or those who have taken a career break.
Cons:
- It is focused on the impact of an individual researcher dependent on number of outputs and length of career, rather than a consistent view considering the multi-faceted aspects of research.
- The h-index treats all citations equally, regardless of the size, significance, or impact of the publication.
- It is not recommended as an indicator of research performance because of its bias against early career researchers and those who have had career breaks.
- The h-index is also meaningless without context within the author's discipline. Different fields have varying citation practices and publication rates. Comparing h-indices across fields can be misleading as what constitutes a high or low index can differ significantly.
- There is too much temptation to pick and choose h-indices from different sources to select the highest one. h-indices can differ significantly between different sources due to their different datasets – there is no such thing as a definitive h-index.
- The h-index does not consider negative citations or retractions, which could provide a more nuanced view of a researcher's impact.
Field Weighted Citation Impact (FCWI, Scopus)
FWCI is the number of citations received by a document, divided by the expected number of citations for similar documents. An FWCI of 1 means that the output performs as expected for the global average; an FWCI of 1.44 means 44% more cited than expected. FWCI is a better metric because it measures the citation impact of the output itself, not the journal in which it is published and compares like-with-like (outputs of the same age and type as classed by Scopus).
Pros:
- It measures the citation impact of the output itself, not the journal in which it is published.
- It attempts to compare like-with-like by comparing an output's citations with those of other outputs of the same age and type classed by Scopus as being in the main subject area. This side-steps the problems inherent in using one measure to compare articles in different disciplines - an FWCI of 1.44 is just as good in History as in Oncology.
Cons:
- It could be seen as disadvantaging work that is purposefully multi- and cross-disciplinary.
- In disciplines with lower average citations, one very highly cited paper can skew the average for all other papers.
Field Citation Ratio (FCR, Dimensions)
FCR is similar to FWCI, but sources data from the Dimensions database.
Pros:
- The data set Dimension uses is much larger than the Scopus one
Cons:
- Citation distributions within journals are highly skewed.
- Is field-specific.
- can be manipulated
- Data used to calculate FCR are not transparent nor openly available.
Publications in top percentiles of cited publications - field-weighted (Scopus)
The number of publications of a selected entity that are highly cited, having reached a particular threshold of citations received. This metric counts and ranks the number of citations for all outputs worldwide covered by the Scopus dataset for its publication year. Data are more robust as the sample size increases (comparing a unit to one of a similar size is more meaningful than comparing one researcher to another) and are normalised by field.
Pros:
- Measures include the top 1%, 5%, 10% or 25% of most cited documents worldwide.
- Should be field-weighted from within SciVal to benchmark groups of researchers.
- Should use 'percentage of papers in top percentile(s)' rather than 'total value of papers in top percentile(s)' when benchmarking entities of different sizes. Counts and ranks the number of citations for all outputs worldwide covered by the Scopus dataset.
- Percentile boundaries are calculated for each year, meaning an output is compared to the percentile boundaries for its publication year.
- Can be used to distinguish between entities where other metrics such as number of outputs or citations per output are similar.
Cons:
- Data are more robust as the sample size increases; comparing a unit to one of a similar size is more meaningful than comparing one researcher to another.
Altmetrics
Alternative metrics ("altmetrics") captures online attention surrounding academic content e.g. Twitter, Facebook and Social Media activity; mentions in Policy documents and registered Patents; Media coverage etc.
They offer useful information about impact outside of scholarly publishing and can serve as early indicators of possible intentions to cite publications. They are a useful tool that can help create success narrative around research impact.
The University has a subscription to altmetric.com and the Open Research Team provided training on the use of the Portal as part of the Researcher KnowHow.
Pros:
- Can give an indication of the wider impact of outputs, tracking their use in policy documents, news items, and so on.
- Can provide an early indicator of the likely impact of a paper, before it has had time to be cited in the future - there's a correlation between number of Mendeley readers saving a paper (which can be tracked via Altmetric Explorer) and eventual number of citations.
- Normalises the different types of attention to give more weight to policies and patents compared to social media posts.
Cons:
- Open to being artificially influenced. Altmetric Explorer will discard where someone has repeatedly tweeted about research for example, but may not be sophisticated enough to detect where multiple accounts have tweeted a DOI just to increase an Altmetric score.
Metrics providers
The most commonly used metric providers are:
- Web of Science owned by Clarivate Analytics
- Scopus by Elsevier
- Google Scholar
- Dimensions
The source of metrics should never be mixed and matched for comparison purposes, e.g. do not compare citation count taken from Web of science with citation count taken from Scopus. The data source differs, and therefore, the final figure may also differ!
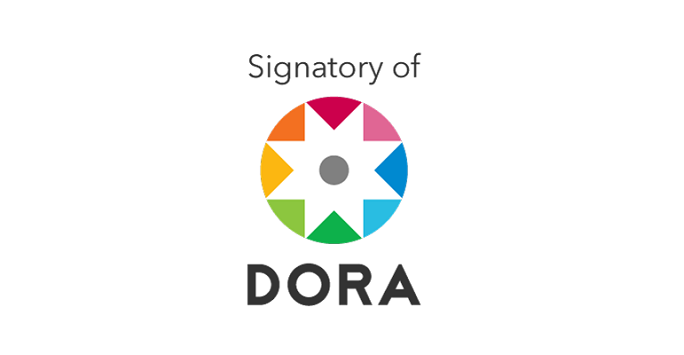
Declaration on Research Assessment
The University of Liverpool is a signatory to the San Francisco Declaration on Research Assessment (DORA) which outlines several recommendations around the use of metrics. This means that as an institution we have committed to avoiding the use of journal-based metrics as surrogate measures of the quality of research in decision-making for funding, appointing and promoting staff, and to assess contributions of individual researchers, and instead assess research based on its own merits.
Back to: Open Research