LIV.DAT welcomes new Fellow
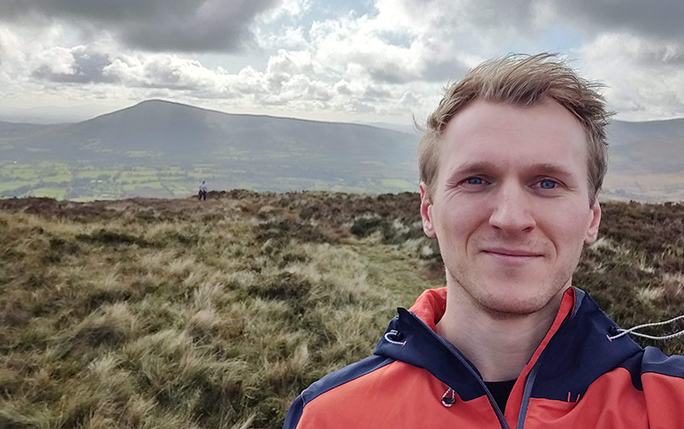
The LIV.DAT Centre for Doctoral Training would like to welcome Dr Egidijus Kukstas, who joined LIV.DAT as a Fellow in September 2020.
At LIV.DAT PhD students are offered a comprehensive training in data science alongside projects in the field of accelerators. Building on his expertise in simulation, data handling and analysis, Egidijus provides support and training to these students. His activities range from tasks such as running specialised software on High Performance Computing (HPC) machines, optimising code to take full advantage of the available resources to more hands-on aspects such as model training and interpretation of results.
Egidijus also carries out his own research into machine learning/AI and how these tools can be applied to accelerator science done at the institute. Beam monitoring and diagnostics are big parts of optimal accelerator operation; there is good potential for machine learning/AI algorithms to be used in event classification and forecasting – something which has historically been done by scientists and technicians. Furthermore, he will help establish and develop industry partnerships.
Egidijus Kukstas graduated with a MSci degree in Physics with Astrophysics from the University of Glasgow in 2016. Whilst at Glasgow, Egidijus gained research experience through a number of summer projects. In the latter years of the programme, his interests migrated towards the field of cosmolgy. His masters project assessed the feasibility of an alternative model of the Universe as a way of explaining Dark Energy. It involved subjecting the non-standard 'Timescape' model to internal consistency checks as well as parameter estimation tests.
In 2020, Egidijus was awarded his PhD from Liverpool John Moores University. His thesis focussed on galaxy evolution in dense environments; specifically, how environmental processes can 'quench' star formation in satellite galaxies. He adapted the method of 'cross-correlations' to be used to quantify the level of influence that environment exerts on galaxy star-formation activity. Egidijus computed two-point statistics encapsulating the cross-correlation in real observations and compared them against equivalent synthetic observations from cosmological hydrodynamical simulations. The use of this technique allows for galaxy environment to be studied without the need to find individual galaxy groups or clusters.
A warm welcome to Dr Egidijus Kukstas!