Call for Papers: RGS-IBG 2018 Annual Conference
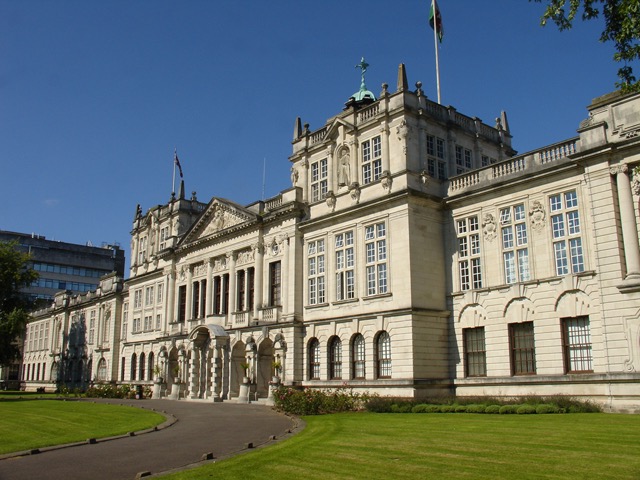
The potential of machine learning to revolutionise scientific study across multiple disciplines has been touted by many commentators (e.g. Jordan & Mitchell 2015). Despite this growing interest, social science and Geography in particular have not shown much engagement with the field. Applications so far tend to focus on prediction and optimisation issues, such as improving Google’s Translate service, paying less attention to the inferential perspective, which is traditionally more aligned with social scientists.
Despite this apparent lack of engagement, there have been some attempts in the past at bridging artificial intelligence with Geography (e.g. Openshaw, 1997; Couclelis, 1998) and there are clear opportunities and potential for synergies in both directions. Machine learning offers new opportunities to process data with high dimensionality making them adept at analysing the rich detail collected across thousands of variables. The iterative ‘learning’ of data insights of algorithms may also detect underlying patterns not seen by humans. These strengths offer potential to contribute strongly to current Geographical analyses. At the same time, most machine learning algorithms are completely aspatial in the sense they ignore the location of observations. Including information on location is likely to improve predictive power and thus the main goal of most of this class of algorithms.
The aim of the session is to start a dialogue within Geography to evaluate and understand the potential applications of machine learning to enhance current Geographical lines of enquiry, as well as the ways in which Geography may contribute to the field of machine learning.
We invite contributions that explore:
- Applications of machine learning to enhance our understanding of a Geographical topic.
- Geographical approaches that ‘open up’ the ‘black box’ nature of algorithms.
- The evaluation of algorithms to assess their relative strengths and weaknesses.
- The extensions of algorithms to deal with the spatial dimension explicitly.
Please can you send a title and abstract (300 words max) to either Mark Green (mark.green@liverpool.ac.uk) or Dani Arribas-Bel (darribas@liverpool.ac.uk) by 4pm, Thursday 15th Feb. We strongly encourage applications from Post-graduate and early career researchers.
Machine Learning in Geography. Geography in Machine Learning.Sponsored by Quantitative Methods Research Group
Mark A Green and Daniel Arribas-Bel Department of Geography & Planning, University of Liverpool, UK.