Virtual seminar on merging deep learning with physical models for the analysis of cosmological surveys
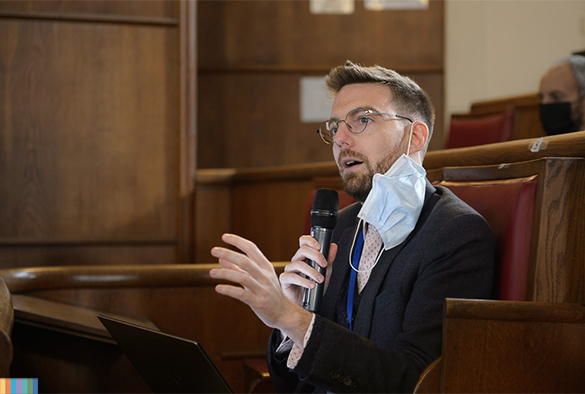
Our Liverpool Virtual Seminars on Data Intensive Science – Autumn / Winter 2022 will start on Monday 10th October 2022 at 15:00 BST. The seminar will be given by Dr Francois Lanusse, a CNRS researcher in observational cosmology and machine learning at the Astrophysics Department of CEA Paris-Saclay (France), who will present “Merging deep learning with physical models for the analysis of cosmological surveys”.
Seminars in this series cover R&D outside of our Data Science Centre’s core research areas and give an insight into cutting edge research in this area. At the end of the talk there will be a Q&A session with the speaker.
About the talk
As we move towards the next generation of cosmological surveys, our field is facing new and outstanding challenges at all levels of scientific analysis, from pixel-level data reduction to cosmological inference. As powerful as Deep Learning (DL) has proven to be in recent years, in most cases a DL approach alone proves to be insufficient to meet these challenges and is typically plagued by issues including robustness to covariate shifts, interpretability, and proper uncertainty quantification, impeding their exploitation in scientific analysis.
In this talk, Dr Lanusse will instead advocate for a unified approach merging the robustness and interpretability of physical models, the proper uncertainty quantification provided by a Bayesian framework, and the inference methodologies and computational frameworks brought about by the Deep Learning revolution.
In particular, we will see how deep generative models can be embedded within principled physical Bayesian modelling to solve a number of astronomical ill-posed inverse problems ranging from de-blending galaxy images, all the way to inferring the distribution of dark matter from weak gravitational lensing measurements. Dr Lanusse will also illustrate how the same generative modelling techniques can alleviate the need for analytic likelihoods in cosmological inference, enabling instead Simulation-Based Inference in which the physical model is implemented in the form of a numerical simulator. And finally, he will highlight the power of the computational frameworks initially developed for Deep Learning when applied to physical modelling, with applications ranging from speeding up cosmological MCMCs to performing inference over the initial conditions of N-body simulations.
About the speaker
Dr Lanusse is a CNRS researcher in observational cosmology and machine learning at the Astrophysics Department of CEA Paris-Saclay (France). He received his PhD in cosmology and inverse problems in 2015 in Paris, and further developed an interdisciplinary expertise in Deep Learning for cosmology as a postdoctoral researcher at Carnegie Mellon University (2015-2018) and UC Berkeley (2018-2019) through multiple collaborations with their respective Machine Learning and Statistics Departments. He is now broadly interested in developing scientific applications of state of the art Deep Learning techniques, by combining concepts of Bayesian inference, deep neural networks, and physical forward modelling.
How to attend
Participation is free, but you need to register to attend this and other webinars in the series. Once registered, you will receive the Zoom connection details on the morning of the online seminar.
For more information and how to register please visit: https://indico.ph.liv.ac.uk/e/data_science_seminars
The seminar details
Speaker: Dr Francois Lanusse (CNRS researcher in observational cosmology and machine learning at the Astrophysics Department of CEA Paris-Saclay (France))
Seminar title: “Merging deep learning with physical models for the analysis of cosmological surveys”
Date/Time: Monday 10th October at 15:00 BST