Alberto Acuto’s industry placement at IQBlade
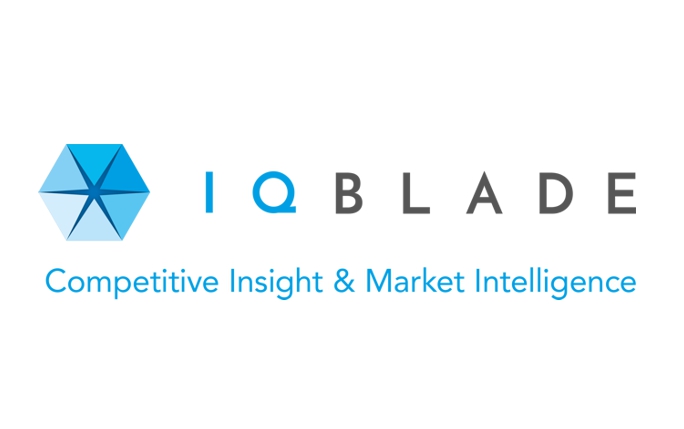
A key feature of the LIV.DAT CDT is the industry placement during which the student is placed in a data-intensive company for a 6 month period to apply skills obtained during their big data training within an industrial setting. LJMU-based LIV.DAT student Alberto Acuto has recently completed his placement at IQBlade, a company based at Liverpool Science Park, where he worked on the development of tech company classification algorithms.
The company IQBlade, which was founded in 2016, has a team of data scientists and tech channel experts who work together as part of their process to help companies who want to find new partners, clients and network growth from a data-driven perspective in a self-contained platform. To remark the success in their approach, they have been acquired by multinational tech company Tech-Data in 2019.
The strength of this platform is the size and quality of the data available in the database with UK-based companies which is now expanding to include European based companies. The platform provides many insights into, for example, economics values, size, social media accounts and yearly growth. The rate at which this database is growing, as well as the continued need of improving search results for clients, has highlighted a number of technical challenges in the big data realm. An example is the establishment of industrial classification of companies within the database.
Liverpool Science Park. (Copyright: Liverpool Knowledge Quarter)
The project that Alberto worked on was focussed on building a classification algorithm based on the descriptive features obtained from text to classify similar types of companies. The basis for this project was established when the need increased to classify a growing number, which currently stands at over four hundred thousand, of ‘not-yet-classified’ listed companies in a smarter and quicker fashion. The idea behind the project was to use data available on the database to create models to make predictions on the unlabelled data.
The project can be briefly explained as follows:
- Build calibrated models on already labelled companies using the text provided by company introduction and text from websites.
- Analyse the unknown labelled company information such as name, URL, general industrial classification and the text and score the results.
- Process the result in a decision matrix procedure to obtain a classification label for the company.
The classification of the companies is a fundamental, but at the same time complex, task. The information gathered might not always be complete. For instance, companies labelled as ISV (Independent Software Vendors) often have the same key features as vendors or resellers, so text-based features can only provide a generic identification as part of the classification. In fact a further step that can be taken to provide a more specific classification is to include economics metrics in the labelling and feed it into the decision matrix.
Alberto said about his placement: “The task was challenging for several reasons, first of all coming from a different background and the lack of basic knowledge was something that I had to deal with at the beginning. Luckily the team helped me out a lot in getting to the right pace to proceed in the work. That was the first hands-on experience in text mining (Natural language processing) and database queries and handling so I was really curious to experiment with those new tools. It was really interesting to see and apply what I have learnt in the last few years and to finally make the most out of that knowledge. The last six months have been challenging and interesting and I grew up a lot in many different aspects.”