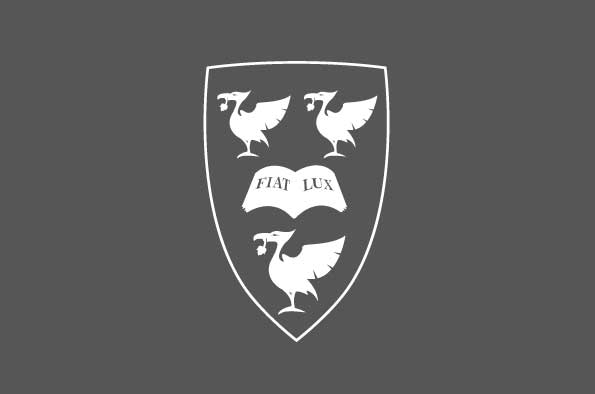
Women in Technology Series: Gender preserving debiasing for pre-trained word embedding
- Shagufta Scanlon
- Suitable for: Staff and students in the School, Faculty or University with an interest in the subject or gender equality
- Admission: Free https://www.eventbrite.co.uk/e/women-in-technology-lecture-series-professor-danushka-bollegala-tickets-75121679967
Add this event to my calendar
Click on "Create a calendar file" and your browser will download a .ics file for this event.
Microsoft Outlook: Download the file, double-click it to open it in Outlook, then click on "Save & Close" to save it to your calendar. If that doesn't work go into Outlook, click on the File tab, then on Open & Export, then Open Calendar. Select your .ics file then click on "Save & Close".
Google Calendar: download the file, then go into your calendar. On the left where it says "Other calendars" click on the arrow icon and then click on Import calendar. Click on Browse and select the .ics file, then click on Import.
Apple Calendar: The file may open automatically with an option to save it to your calendar. If not, download the file, then you can either drag it to Calendar or import the file by going to File >Import > Import and choosing the .ics file.
Authors: Masahiro Kaneko (Tokyo Metropolitan University, Japan) and Danushka Bollegala (University of Liverpool, UK)
Abstract: Word embeddings learnt from massive text collections have demonstrated significant levels of discriminative biases such as gender, racial or ethnic biases, which in turn bias the down-stream NLP applications that use those word embeddings. Taking gender-bias as a working example, we propose a debiasing method that preserves non-discriminative gender-related information, while removing stereotypical discriminative gender biases from pre-trained word embeddings. Specifically, we consider four types of information: feminine, masculine, gender-neutral and stereotypical, which represent the relationship between gender vs. bias, and propose a debiasing method that (a) preserves the gender-related information in feminine and masculine words, (b) preserves the neutrality in gender-neutral words, and (c) removes the biases from stereotypical words. Experimental results on several previously proposed benchmark datasets show that our proposed method can debias pre-trained word embeddings better than existing SoTA methods proposed for debiasing word embeddings while preserving gender-related but non-discriminative information.
Discussions: Following the talk, the audience will have the opportunity to direct questions to the speaker and also to members of the discussion panel, the confirmed panel members are as follows:
Prof. Simon Maskell (Electrical Engineering and Electronics)
Dr. Rebecca Davnall (Philosophy)
Dr. Zainab Hussain (Health Sciences), Chair of BAME Staff Network