Magnetic Refrigeration: Combining Experiment and Machine Learning to Make a Cool Choice
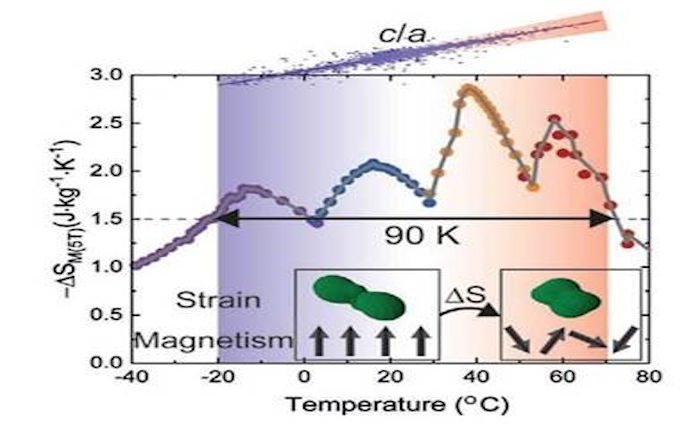
In February, our paper titled “Chemically Controllable Magnetic Transition Temperature and
Magneto-Elastic Coupling in MnZnSb Compounds” was published in the journal Advanced Functional Materials. This work was written by PhD student Phil Murgatroyd, who has just submitted his thesis, and supervised by Dr Jonathan Alaria. The work was a collaboration between condensed matter physicists and solid-state chemists at the University of Liverpool, computer scientists in the Leverhulme Research Centre for Functional Materials Design, crystallographic experts from the Institut Laue-Langevin, and condensed matter physicists from CRISMAT Caen.
As the threat of the climate crisis becomes more acute, the importance of research into alternative technologies becomes greater. Society understands the harm CO2 emissions inflict upon the environment and the importance of minimising such emissions. However, it is less well known that cooling systems, based upon the vapor-compression cycle, are estimated to be responsible for ≈8% of global CO2 emissions. Magnetic refrigeration, based upon the magnetocaloric effect, is an environmentally friendly alternative. Unfortunately, the discovery of magnetocaloric materials is challenging, and requires computational proxies and machine learning algorithms to guide exploratory synthesis.
We performed a theoretical and experimental study upon a family of ferromagnetic materials belonging to the Mn-based PbFCl alloys. Through a combination of computational and experimental methods we found that MnZnSb is a potential room temperature magnetocaloric material with favourable performance metrics. The origin of the magnetocaloric effect is a large entropy change through the ferromagnetic transition arising from the coupling of magnetic moment formation and lattice strain release. This is known as “magneto-elastic coupling”. Furthermore, we report that the transition temperature of the system can be controlled and optimised for application purposes through simple chemical substitution. We report that the chemical substitution altered the crystallographic c/a parameter which led to a change in the transition temperature. Due to the relationship between transition temperature and the c/a parameter, a machine learning algorithm was constructed to predict the c/a ratio of PbFCl alloys. It is our hope that this machine learning algorithm will inspire future exploratory synthesis of novel materials with desirable physical properties to tackle the climate crisis and other problems that society faces.